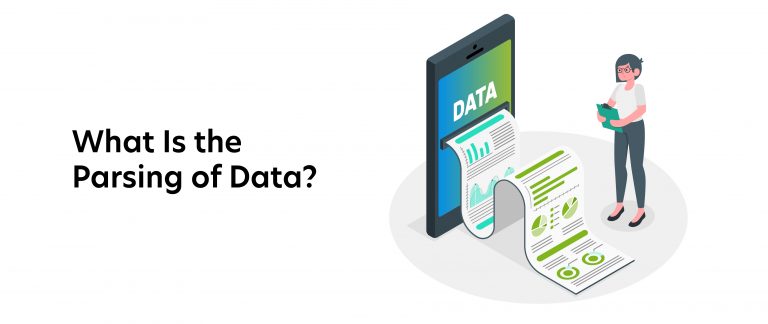
If you deal with a large amount of data, you must have come across the term data parsing. After the extraction of data from the web, some work has to be done on the extracted data to make it into a format that is more readable and better for analysis.
This is the definition in simple terms, but what is data parsing on a wider scale?
In this article, we would cover everything about the parsing of data including the algorithms and technology.
Post Quick Links
Jump straight to the section of the post you want to read:
WHAT IS DATA PARSING?
Data parsing is the next step done immediately after the extraction of data. It is the process of converting received data into a different format that is more acceptable and readable. Since most data available online is in HTML format which is not easily readable and understood, it’s, therefore, necessary to convert the data into a format that can be understood and read.
WHAT DOES A PARSER DO?
With parsing, not every string of data gets worked on and converted, but only the necessary string gets converted. A good parser can differentiate the information in the HTML string that is needed from the rest, based on the parser’s rules and codes. The string of data that is picked and worked on would be converted into CSV, JSON, or even a table.
The parser itself isn’t tied to any particular format. Every conversion that takes place is based on how the parser was built.
Parsers can be used in various ways including;
1 . Scripting languages
-
HTML and XML
-
Interactive data language and object definition language
-
Java language and others for programming
-
Modeling languages
-
SQL and other database languages
-
HTTP, HTTPS, and other internet protocols
PARSING BEHAVIOR
Data parsing takes place in two steps;
1 . Primary: this step involves the allocation and population of structures of collected data before execution.
- Secondary: in the secondary step, the allocated data gets executed based on the parser’s code and data in the structures.
WHY IS DATA PARSING NECESSARY?
The structure of data plays a role in its purpose. Sometimes, the structure of data makes the data unfit for use where it would be needed. For example, the data capture system lacks fields for the input of distinct pieces of information, each with their use. And so the user has to enter different pieces of information into a single text field, or input information into the wrong text boxes as they lack specific places. This data needs to be moved to a different system with a different data structure for proper working. Duplicates that may exist need to be removed but this would be difficult to do because of the data structure.
Sometimes, it may not be the structure of the data that makes it use inconvenient, but due to error. This is the case if, for example, users are not skilled enough to enter all necessary information leading to issues like entering names as data cheats instead of real names where applicable. The application may also display fields in the wrong way, causing users to enter information in the wrong fields. Users could also enter records that are wrong in more than one field and it becomes hard to detect. An instance of this is when inaccurate data is entered in multiple records that represent the same entity, or when accurate data is entered but in the wrong field.
All of these problems cause poor data quality ultimately and could harm the business. So to avoid the downfalls of inaccurate data, these problems need to be fixed before the data is used.
WHICH IS BETTER; TO BUILD OR TO BUY A DATA PARSER?
This is a frequently asked question especially among those who are just getting into the world of parsing. It’s cheaper if you build your parser, but apart from the cost, they are a lot of other considerations to be taken into account.
1 . BUILDING A DATA PARSER
When building your parser, you stand to benefit from the following;
1 . The parser would be made specific to your parsing needs
- You have control over the updates and maintenance of your parser
- You will spend less to build your parser
The good of building your parser doesn’t come without some downsides. They include;
1 . When building your parser, you will need to hire a team to help with the process
- Maintenance is necessary and this will incur expenses on you
- You will need to purchase a server that is suitable for your parsing needs
- Since you would be in control, you will always have to be available for decision making, planning, and testing to come out with something good.
The benefits of building a parser are obvious, but due to the resources and time that would be required, it may not be the most suitable approach to obtaining your parser.
2. BUYING A DATA PARSER
If you have considered building your parser and it doesn’t sit well with you, you could buy one instead. The following are the benefits of buying a parser:
1 . Since everything from maintaining and the building would be taken care of, you wouldn’t need to pay for human resources
- Decisions concerning the parser would be made faster and would be way better as those in charge of maintaining the parser are skilled professionals who know what they are doing.
- Built for the public, it is more likely to be close to perfection. So the chances of having issues would be slim.
- You will have more time to yourself as the decisions and testing would be done for you by the experts.
Its downsides however include;
1 . Since everything would be done for you, the cost would be more
- Your control over the function of the parser would be limited
ALGORITHMS AND TERMINOLOGY INVOLVED IN PARSING
In this part, we would be explaining the concepts and algorithms that are involved in data parsing, so that you can have a better understanding of what goes on. The three parts that would be dealt with here are;
- Components and terms of a data parser
- Grammars
- Algorithms
1. COMPONENTS AND TERMS OF A DATA PARSER
A. REGULAR EXPRESSIONS
Regular expressions are a sequence of characters that have a pattern. Even though they are popularly regarded as not fit for parsing, they can be used for parsing simple input. The misconception is due to the errors that arise when regular expressions are used to parse everything including what they are not meant for. When that is done, it ends with a series of fragile regular expressions that ae hacked together.
Regular expressions can also be used to parse some simple programming languages. Not all languages can be parsed using regular expressions and the languages that can be used ae referred to as regular languages. Regular languages can also be parsed using a finite state machine and as this is also powerful, it can be used to implement lexers.
While you can define a regular language using a series of regular expressions, more complex languages require something more. As a rule, if a grammar of a language has elements that are either recursive or nested, it’s not a regular language. An instance is HTML. It can contain a random number of tags inside another tag and so it can’t be said to be a regular language. By extension, it cannot be parsed using only regular expressions no matter how skilled the parser is.
Regular Expressions in Grammars
Since most programmers are familiar with regular expressions, they are often used to define language grammar. Their syntax is more precisely used to define the rules of a parser or lexer. For instance, the Kleene star is applied in a rule as an indicator that a particular element can be present for any number of times starting from zero to infinity.
The rule is not the same as the implementation of a lexer or a parser. You can make use of the regular expression engine of your language to implement a lexer. For even better performance, the regular expressions in the grammar ae converted to a finite state machine.
B. STRUCTURE OF A-PARSER
A complete parser usually has two parts; the lexer which is also known as the scanner or tokenizer, and the proper parser. The parser doesn’t work directly on the text but only on the output of the lexer, and so it needs the lexer. Some parsers however do not have a separate lexer but rather combine the lexer and the parser. These are referred to as scanners parsers.
The lexer first scans the input and then produces the matching tokens after which the parser scans the tokens and gives the parsing result.
Scannerless Parsers
Scannerless parsers are different in the way they operate as they act directly on the original text instead of tokens produced by a lexer. So a scannerless parser acts both as a lexer and a parser.
It’s not important to define the grammar, but for purposes of debugging, you would need to know if a parser is scannerless or not.
C. GRAMMAR
Grammar rules that describe a language syntactically. A grammar describes a language and this is only applicable to the syntax and not the semantics. This implies that grammar defines the structure of language and not it's meaning. The input must be checked by some other means to be sure it's correct.
For instance, imagine a grammar for the language shown in the paragraph definition is to be defined;
HELLO: “Hello”
NAME: [a-zA-z] +
Greeting: HELLO NAME
The acceptable input by the grammar is “Hello Michael” and “Hello Programming”. Either way, they are correct. Since “Programming” isn’t a name however, it’s wrong semantically.
ANATOMY OF A GRAMMAR
There are some commonly used formats used to describe grammar and an example is the Backus-Naur Form (BNF). This format has variants, one of which is the Extended Backus-Naur Form and its advantage is its simplicity in denoting repetition. Another variant of BNF is the Augmented Backus-Naur Form. It’s useful in the description of bidirectional communications protocols. When using Backus-Naur grammar, a typical rule has the following representation;
<symbol> : := _expression_
The
Terminal symbols are the symbols that don’t appear as
A rule in the technical sense defines a transformation between the nonterminal set of elements and the nonterminal and terminal sets of elements on the right side. It is also known as production rule.
TYPES OF GRAMMARS
In parsing, two types of grammar exist basically. They are regular grammars and context-free grammars. Normally, regular grammar would be used to define a regular language and so on, but the recent kind of grammar known as Parsing Expression Grammar (PEG), can be used to also define context-free language since its as powerful as context-free grammars. The difference between the two types lies in the notation and the way the rules are interpreted.
In terms of complexity, regular languages are simpler than context-free languages and they can both be distinguished by the _expression_ of a regular grammar. This implies that the right side could be only one of the following;
- A single terminal symbol
- The empty string
- A terminal symbol followed by a nonterminal one
This is easier in theory than in practice as it’s hard to check because a tool could allow the use of more terminal symbols in a single definition and then transform the expression in an appropriate series of expressions that al belong to one of the above-mentioned cases.
So even if you write an expression that isn’t compatible with a regular language, the expression would be transformed into the proper form.
D. LEXER
A lexer function to transform a sequence of characters present in a sequence of tokens thus they are also called scanners or tokenizers. Lexers are important in parsing as they transform the input into a form that is better manipulated by the parser at the later stage of the process. Normally, lexers are easier to write than parsers although in some cases, both are equally complex.
An important function of lexers is dealing with whitespace. You would need to discard the whitespace using lexer because its presence would have the parser checking for it between every token and it’s an annoying process. You can’t always discard the whitespace however as its relevance in some cases, for example in python where whitespace is used to identify a block of code. Even in such cases, the lexer is used to distinguish the relevant whitespace from the irrelevant ones before parsing.
WHERE THE FUNCTION OF LEXER ENDS AND THE PARSER BEGINS
In most cases, lexers ae used together with parsers so the division between the two can be difficult to make most times. This is because after parsing, the result should be one that is relevant to the program. So in the end you care only about the method of parsing that suits you even if there are many ways to parse data.
PARSER
In the broad sense, a data parser is a software that performs the entire process of parsing, but being specific, the parser analyses the tokens that are produced by the lexer. This implies that the parser handles the most important and difficult part of parsing and the lexer assists in the process.
The output of a parser is usually an organized structure of its code and it comes in the form of a tree. The tree could be a parse tree or an abstract syntax tree and the differences between each is in the way they represent the code and the intermediate elements defined by the parser. A tree is chosen because it makes it more convenient to work with the code at different levels.
Syntactic Correctness vs Semantic Correctness
Parsers are important in compilers or interpreters but are not restricted to these as they can also be a part of other software. A parser can be used to check the syntactic correctness of code, but in checking the semantic validity, the compiler would have to use the output.
In the following example, the code is syntactically correct, but incorrect semantically.
int x = 10
int sum = x + y
since the (y) variable is not defined, the program would fail if the code is executed. The parser wouldn’t know this as it only looks at the code’s structure rather than keep track of variables. A compiler on the other hand goes through the parse tree and keeps track of all the variables that are defined the first time. It goes over the tree a second time to cross-check that the variables that are used are properly defined.
SCANNERLESS PARSER
A scannerless parser is also called a lexerless parser and it performs tokenization and parsing all in one step. If the distinction between lexer and parser is not necessary, or difficult, it’s better to make use of a scannerless parser.
PROBLEMS WITH PARSING REAL PROGRAMMING LANGUAGES
Theoretically, parsing is meant to deal with real-life programming languages, but this is an issue due to some challenges.
Context-sensitive parts
Even though parsing tools are meant to handle context-free languages, the languages are context-sensitive in some cases and it becomes a problem. An example of a context-sensitive element is soft keywords (strings of elements that could act as keywords in certain places and also act as identifiers in others).
Whitespace
Whitespace is very important in some programming languages like python, where the indentation present on a statement indicates that it belongs to a certain block of code.
Even though whitespace is relevant in python, it is also irrelevant in some places such as the space between words or keywords. The problem is the indentation and the easiest way to deal with this is to check the indentation at the beginning of a line and transform it into the proper token.
Multiple Syntaxes
Another issue with using real programming languages in parsing in that the language might contain sections of code that have different syntaxes. The most common example of this is the C or C++ preprocessor which is a complicated language on its own it’s and can appear randomly inside any C code.
In the case of annotations, you can more easily deal with them, and they are present in many programming languages. They can be used to process the code before it gets to the compiler and can command the annotation processor to transform the code in a specific way before the annotated one. Since they only appear in specific places, they are easier to deal with.
Dangling Else
This problem is common in data parsing especially those that are linked to the if-then-else statement. Else clause is an optional one and so the if statement could mean anything. For example;
If one
Then if two
Then two
Else ???
In the example, it isn’t clear if the else is for the first or second if.
In handling the problem, the method used conventionally involves associating the else to the nearest statement of if and doing this makes the parsing context-sensitive.
PARSE TREE AND ABSTRACT SYNTAX TREE
These two terms are closely related and sometimes used interchangeably. Both of them are similar as they are both trees and have a root with nodes that represents the entire source code. The root has subsequent nodes, which themselves contain subtrees that represent smaller portions of code until the emergence of single tokens.
The difference between the two is in their levels of abstraction. In a parse tree, you may find all the tokens that are in the program and also a set of intermediate rules. But in an abstract syntax tree, only the relevant information that helps to understand the code remains.
A parse tree represents the code that is closer to the concrete syntax. It shows different levels of details of the parsing process.
// lexer
PLUS: ‘+’
WORD PLUS: ‘plus’
NUMBER: [0-9]+
// parser
// the pipe | symbol indicates an alternative between the two
Sum: NUMBER (PLUS | WORD_PLUS) NUMBER
In the grammar, a sum can be determined with the plus (+) symbol or the use of the string plus.
When parsing the following code;
10 plus 21
The resulting parse and abstract syntax tree would be;
The indication of the specific operator is absent in the AST, and the only remaining in the operation yet to be performed. The specific operator is an intermediate rule.
GRAMMARS
Grammars are rules that are used to describe a language. Grammar has several elements that need to be given attention as grammar can also be used to define duties or to execute codes.
GRAMMAR ISSUES
Missing Token
In some types of grammar, only a few tokens are defined. Example;
NAME : [a-zA-Z]+
Greeting : “HELLO” NAME
The token “HELLO” isn’t defined and this usually happens because some tools generate the corresponding tokens for a string to save time.
Left-recursive Rules
An important feature of parsers is the support it should have for left-recursive rules. This implies that a rule should begin with a self-reference. This reference could be indirect and appear in another rule that is referenced by the first one.
For example, in arithmetic operations, an addition could be described as two expressions divided by the plus symbol, but the quantity of the additions could also be other additions.
Addition : expression ‘+’
expression
Multiplication : expression ‘+’
expression
// an expression could be an addition or a multiplication or even a number expression : multiplication | addition | [0-9]+
In the above example, the expression has an indirect reference to itself through the rules of addition and multiplication.
The description is also similar to multiple additions like 5 + 4 + 3. It’s so because it can also be interpreted as expression (5) (‘+’) expression (4+3. The rule of addition here is that the first expression corresponds to the option [0-9]+ and the second one is also an addition. 4 + 3 can also be divided into its two constituent parts;
Expression (4) (‘+’)
Expression (3)
The rule of addition here is that both expressions correspond to the option [0-9]+
Since left-recursive rules may not be used with some parser generators, the other option would be a long chain of expressions that take care of the most important operations.
Predicates
Predicates are rules that match only under the required conditions. They are also called syntactic or semantic predicates. The required condition is defined using a code that is supported by the tool that the grammar was written for.
The advantage of predicates is that they permit some form of context-sensitive parsing which is unavoidable in matching certain elements sometimes. For example, they can be used to check if the sequence of characters that define a soft keyword is in the right position where it would ultimately be a keyword. Its disadvantage is that it can slow down the parsing process and also make grammar dependent on the programming language the condition is expressed in.
Embedded Actions
Embed actions single out codes that are executed once the rule is matched. Their disadvantage is that grammar is more difficult to read because the rules are surrounded by codes. Just like predicates, they also break the division between a language describing grammar, and the code that manipulates the parsing results.
Embedded actions are used more by less sophisticated parsing generators as the only way codes can easily get executed once a node is matched. With parser generators, the only way would be to access the tree and execute the right code yourself. With more advanced tools, you can execute arbitrary code using the visitor pattern when it's needed.
Actions can also help to add certain tokens or change the generated tree and this could be the only option in dealing with complicated programming languages like C.
FORMATS
Concerning grammar, there are two main types of formats; BNF and all its variants, and PEG. Many tools also implement their specific variations of the formats, while some tools use custom formats completely. Custom format is made of three parts; options with the custom code, and then the lexer section which ends in the parser section.
Since BNF formats are the foundation of a context-free grammar, it could also be identified as CFG format.
BACKUS-NAUR FORM AND ITS VARIATIONS
The BNF is a very successful format and is the basis for the creation of PEG. Since its very simple, it’s not mostly used in its based form but rather in the form of a more powerful variant. In the below example, the importance of BNF variants can be seen;
<letter> ::= "a" | "b" | "c" | "d" | "e" | "f" | "g" | "h" | "i" | "j" | "k" | "l" | "m" | "n" | "o" | "p" | "q" | "r" | "s" | "t" | "u" | "v" | "w" | "x" | "y" | "z"
<digit> ::= "0" | "1" | "2" | "3" | "4" | "5" | "6" | "7" | "8" | "9"
<character> ::= <letter> | <digit>
The
A more difficult issue is that there is no simple way to denote optional elements or existing repetitions, so you would have to depend on Boolean logic and the (|) symbol.
<text> ::= <character> | <text>
<character>
From the rule,
The below example would be the tree parse for “dog”
Other limitations of BNF are that it makes it difficult to make use of empty strings or symbols that are used by the format in the grammar.
Extended Backus-Naur Form
The EBNF was created to solve some of the above-mentioned limitations. It’s the most popular form used in parsing tools and even though tools may differ from the standard notation. EBNF’s notation is cleaner and adopts more operators to deal with optional elements or concatenation.
ABNF
ABNF is short for Augmented BNF and is one of the variants of BNF. It's developed for the main purpose of describing bidirectional communication protocols. The use of ABNF can be as productive as that of EBNF, but due to some of its features, its use is limited to internet protocols.
ABNF also has a different syntax from that of EBNF. For example, the alternative operator is represented with the slash (/). It also has more features than EBNF, for instance, you can define numeric ranges like %x30-39 as [0-9]. It’s also used by designers to include standard character classes-like rules that the final user can use.
PEG
PEG is short for Parsing Expression Grammar. It’s a format that stems from an old grammar format called Top-Down Parsing Language. It’s similar to EBNF and is also used to support widely used variables like character ranges. It’s not entirely similar to EBNF like its use of the formal arrow symbol instead of the normal equals symbol in assignments.
PEG vs CFG
Theoretically, the differences between both formats are limited. PEG is closely likened to the packrat algorithm and that’s it. For example, PEG does not allow left recursion but although the algorithm can be modified to support left recursion, it eliminates the linear time parsing property. PEG parsers are also generally scannerless parsers.
A difference and probably the most important between PEG and CFG is that when ordering choices in PEG, its meaningful unlike in CFG. If there are various valid ways of parsing an input, it will be ambiguous in CFG and an error will return. For example, by providing all the valid results to the developer for sorting out. In PEG, however, ambiguity is eliminated because the first applicable choice will be chosen and so PEG can’t be ambiguous.
The disadvantage is that you have to be extra careful when listing possible alternatives because you would have unexpected consequences in the long run.
ALGORITHMS
Parsing has different algorithms that each have their strong points as well as their weak points, and require updates frequently.
Parsing has two strategies which are; top-down parsing, and bottom-up parsing. Both are defined using the parse tree as generated by the parser.
A top-down parser functions to identify the root of the parse tree first and then goes to the subtrees and then the leaves of the tree. While a bottom-up parser begins from the bottom of the tree and works its way up till the root of the tree.
Initially, it was easier to build top-down parsers even though bottom-top parsers proved to be more powerful. But due to advancement in tech, the situation is now more balanced.
The derivation is related to the strategies and it indicates the order of appearance in which nonterminal elements in the rule on the right are applied to get the nonterminal symbol on the left side. With BNF terms, it can be said to indicate how the elements in –expression_ are used to get
For example, in trying to parse the symbol result as defined in the following grammar;
expr_one = . . // stuff
expr_two = .. // stuff
result = expr_one ‘operator’ expr_two
you can choose to apply the rule for symbol expr_one before expr_two or the other way round. For leftmost derivation, you choose the first option, but you pick the second option for rightmost derivation.
When applying derivation, it’s depth-first or recursively. This means that its first applied to the first expression and then on the intermediate result that is obtained.
COMMON ELEMENTS
These common elements are shared between parsers that are built using top-down and bottom-up strategies.
Lookahead and Backtracking
Lookahead is used to indicate the number of elements that come after the current one and ae taken into consideration for decision making. For example, a parser might check the token that comes next to help in deciding the rule to apply now. After the right rule is matched, the token is consumed but the next one stays in the queue.
Backtracking on the other hand is a technique specific to an algorithm that finds solutions to complex problems by trying out partial solutions and dwelling on the most promising one. If the solution that is being tested fails, the parser then backtracks and tries out another one.
Chart Parsers
Chart parsers could be wither bottom-up, or top-down. They try to avoid backtracking with the use of dynamic programming. Dynamic programming is a method that is used to break down larger problems into smaller ones for easy solving.
The Viterbi algorithm is an example of a common dynamic programming algorithm that chart parser uses. It aims to locate the most likely hidden states through the known sequence of events.
AUTOMATONS
Automatons are abstract machines. Among parsers, Pushdown Automaton (PDA) is common, and among lexers, Deterministic Finite Automation (DFA) is common. PDA is a more powerful and complex machine than DFA.
Since they are used to define abstract machines, they are not directly linked to a real algorithm but are rather used to give a formal description of the level of complexity an algorithm has to be able to deal with.
Since DFA are state machines, the distinction when it comes to lexer is frequently uncertain. This is so because state machines have ready to use libraries and so DFA is implemented most times with a state machine.
Lexing With a Deterministic Finite Automaton
A state machine has many possible states, each with a transition function and an example of a finite-state machine is DFA. The transition functions are responsible for how the machine moves from one state to another in a response to an event. If the machine is used for lexing, the input characters are fed in one at a time until it can build a token.
They are used because they can recognize an exact set of regular languages and so they are as powerful as regular languages. Another reason is that they are a few mathematical methods that can be used to check their properties and manipulate them, and they can work with an online algorithm.
An online algorithm doesn’t require the whole input to work. With a lexer, a token can be recognized as soon as its characters reach the algorithm. You can also transform a set of regular expressions in a DFA and this makes it easy to input the rules in a simple enough way so that developers won’t have any problem working with it. From there you can convert them automatically in a state machine that can work on them efficiently.
TOP-DOWN ALGORITHMS
This is the most popular strategy of the two, and it's applied in several algorithms.
LL PARSER
The LL stands for left-to-right read of the input, leftmost derivation. These parsers are table based and don’t have backtracking, only lookahead. From this, you can see that they don’t depend on any table to make decisions on parsing rules to apply. They find the correct rules to apply by;
The parser first looks at the current token and also the required amount of lookahead tokens
And then it applied the different rules until the right match is found
LL parsers are not specific to a specific algorithm but a class of parsers. So an LL parser can parse LL grammar. LL grammars are defined by the number of lookahead tokens that are required to parse them and the number is indicated between parenthesis next to LL; LL(k).
So it’s safe to say that an LL(k) parser makes use of k tokens of lookahead and so it can parse a grammar that requires k tokens of lookahead to be parsed. LL(k) grammars are used when different algorithms are being compared and it serves as a meter.
Value of LL Grammars
The use of LL grammars from above is because LL parsers are a bit restrictive, and they both have wide use. LL grammars don’t support left-recursive rules and so you can transform any left-recursive grammar and this limitation has an effect on productivity and power.
The loss of productivity is based on the requirement that you have to write the grammar in a specific way and this is time-consuming. Power is limited also because a grammar that may need 1 token of lookahead usually when written using a left-recursive tool may need 2 to 3 tokens of lookahead when it gets written in a non-recursive way.
Loss of productivity can be reduced using an algorithm that transforms a left recursive grammar to a non-recursive one. An example of a tool that can do that is ANTLR but if you are building your own data parser, you would have to do it yourself.
LL(1) and LL(*) are two special types of LL(k) grammars and were the only practical types in the past due to the ease at which parsers could be built for them.
EARLEY PARSER
The Earley parser is a chart kind of parser. This algorithm is likened to CYK, another similar parser but simpler but worse in memory and performance too. An advantage of the Earley algorithm over CYK is that after storing partial results, it also has a feature to predict the rule that is going to be matched next.
Earley parser works basically by dividing a rule into parts. An example is seen below;
// an example grammar
HELLO : “hello”
NAME : [a-zA-Z]+
Greeting HELLO NAME
// Earley parser would break up greeting in the following way
// . HELLO NAME
// HELLO . NAME
// HELLO NAME .
An upside of Earley parser is the guarantee that it can parse all context-free languages, whereas other algorithms like LL or LR can only parse a subset of the languages. For instance, it has no issue with left-recursive grammars. In the general sense, Earley parser is also able to handle non-deterministic and ambiguous grammars.
It can do all of these but at the risk of a bad performance. It however has a linear time performance for normal grammars. The good thing though is that the languages that are parsed by the more traditional algorithms are usually the one of interest.
A side effect to this is that it has no limitations as it forces the developer to write the grammar following a certain format that the parsing can be more efficient. That is to say, building a LL(1) grammar might prove to be difficult for the developer, but the parser on the other hand can apply it better. So Earley makes you work less as the parser does the rest.
In a simple statement, you can say that Earley allows you to make use of grammar that is easier to write even though the performance may not be optimal.
Earley Parser Use Cases
Earley parsers as we have seen are easy to use, but in terms of performance, they are lacking. This up and downside make the algorithm more suitable for use in an educational setting where productivity is more important than speed.
In the first use case, the grammars your user writes work properly but the parser sends random errors at intervals. The errors are actually because of limitations that exist in the algorithm that your users do not understand. So by getting the errors your users are being forced to understand the working of your parser and its unnecessary.
A good case of a situation where the productivity of a parser is more important than its speed is in the use of a parser generator to implement syntax highlighting to help an editor. The editor needs support for many languages and being able to give support quickly might be more important than completing the task quicker.
PACKRAT (PEG)
Packrat and PEG were both invented by the same person and so they are often associated with one another. Packrat parsing has a linear execution time and this is because there is no backtracking. Another reason for its good efficiency is memorization. This is the process of storing partial results while parsing is going on. A drawback however is the amount of memory space that is needed to store the results during the parsing process. If the available memory is not up to that which is required, the linear execution time of the algorithm is lost.
Packrat just as others don’t support left-recursive rules and that is because PEG needs to always choose the first option. Some variants of the algorithm can support direct left-recursive rules but they do this at the price of losing linear complexity.
If necessary, packrat can also perform with an infinite amount of lookahead and this goes on to influence the execution time.
RECURSIVE DESCENT PARSER
This type of parser works with a set of recursive procedures and most times each procedure is to a rule of grammar. And so you can say that the parser structure is a mirror of grammar structure.
A predictive parser is sometimes used as a synonym for a top-down parser, while some others use it to mean a recursive descent parser without backtracks. A recursive descent parser that backtracks is the direct opposite of the second meaning of a predictive parser. So with a backtracking recursive descent parser, whenever a rule in a sequence fails to match the input, it goes back to try another.
Recursive descent parsers do not easily parse left-recursive rules. This is so because the algorithm would repeatedly call the same function over and over. To solve this, you can use tail recursion, and parsers that use this method to solve the repeated calling of a function are called tail-recursive parsers.
Tail recursive parsers are recursions at the end of a function. They are however not used alone but together with transformations of grammar rules and this combination allows recursive-descent parsers to deal with left-recursive rules.
PRATT PARSER
Even though Pratt Parsers are not widely used, those that know its value appreciate it. This algorithm doesn’t rely on grammar but instead on tokens.
Conventionally, top-down parsers work better if there is a prefix that distinguishes different rules. Since this applies to all programming languages, it’s one reason why the Pratt Parser has just a little effect in the world of data parsing.
Pratt algorithm however has great use in expressions. Because of precedence, it’s impossible to understand the input structure just by looking at the order of the tokens. So the algorithm asks for an allocation of precedence value to each token and other functions too that determine actions based on what is to the left and the right side of the token.
PARSER COMBINATOR
This is a higher-order function and it works by accepting parser function as the input and sends the new parser function as the output. A parser function is one that accepts a string and an output as a parse tree.
Even though a parser combinatory is easy to build as they are modular, they are less sophisticated and slower. So they are mostly used for easy parsing tasks or in prototyping. The user of the parser combinatory relies on the creator of the combinator but builds the parser partially by hand.
Parser combinators don’t support left recursive rules just like some other algorithms, but other advanced forms are capable of doing it. Some implementations are also referred to as Monadic Parser Combinator because they rely on the structure of the monad (a functional program). A monad combines functions and also data and it depends on the type of data. The data type is what specifies the various combinations for values.
BOTTOM-UP ALGORITHMS
The success of the bottom-up algorithm is due to the family of many LR parsers. They are however relatively unpopular and that is because they have been known to be difficult to build, even though LR parsers are more powerful than LL(1) grammars.
Shift-reduce algorithms have a two-step function;
Shift: in this step, one token is read from the input and it becomes the new node
Reduce: in this step, the tree that results from the matching of the proper rule is joined with a precedent subtree that already exists
You can say that the shift step deals with reading the input until the completion, and the reduce joins subtrees to build the final parser tree.
CYK PARSER
CYK stands for Cocke-Younger-Kasami. This algorithm has the major disadvantage of requiring that grammars be expressed in Chomsky normal form. This requirement is so because the algorithm depends on the properties of this form to split the input while trying to match all the possibilities. Theoretically, any context-free grammar can be transformed into a matching CNF, but this is not feasible to do by hand.
The algorithm is useful especially for specific problems and an example is the membership problem. It’s used to check for the compatibility of a string with a specific grammar. The algorithm can also be used in processing natural language in looking for the best parsing amongst available options.
LR PARSER
LR is short for the left-to-right read of the input, rightmost derivation. They are examples of bottom-up parsers that can handle deterministic context-free languages linearly concerning time, without using backtracking but with lookahead.
They are compared traditionally with LL parsers, so there is a similarity in the number of lookahead tokens that are necessary to parse a language. An LR(k) parser can parse grammars that require k tokens of lookaheads to be parsed. LR grammars are not as restrictive and are powerful than the matching LL parsers.
In the technical sense, LR grammars are a superset of LL grammars and a significance of this is that only one LR(1) grammar is needed and so (k) is not usually included.
Just like LL parsers, they are also table-based and need two complicated tables. Simply put;
One of the tables determines the action of the parser depending on the current token, its state, and the lookahead sets
The second table tells the parser the next state to move
LR parsers are both powerful and have great performance, but the tables they require are difficult to build by and can grow too large for normal computer languages. So the only way they can be used is through parser generators.
Generalized LR Parser (GLR)
GLR is a powerful variation of LR parsers. They are important to parse nondeterministic and ambiguous grammars. Its power isn’t in the tables which is the same as that of a traditional LR parser but can move to different states. Practically, when an ambiguity exists, it forks a new parser that can handle the particular case.
The worst-case in the complexity of a GLR parser is the same as that of Earley one, even though its performance may be better in the best-case scenario of deterministic grammars. In terms of ease to build, an Earley parser is also easy to build than a GLR parser.
FAQ
1 . HOW TO USE A DATA PARSER?
Every data parser comes with its “how to use manual”. Most of the manuals would require you to be knowledgeable in techs such as python and web scraping.
2. WHAT TOOLS ARE NEEDED FOR DATA PARSING?
After you get the data using web scraping tools, you have options for data parsing. Among data parsing tools are these two common ones; BeautifulSoap, and LXML.
3. WHAT IS DATA SCRAPING?
Data scraping involves the acquisition of a large amount of required data from the web using bots and Proxy.
About the author
Rachael Chapman
A Complete Gamer and a Tech Geek. Brings out all her thoughts and Love in Writing Techie Blogs.
Related Articles
Web Scraping Job Postings: Challenges and Best Solutions
Wouldn’t it be great if we found our ideal jobs in less than 24 hours? Web Scraping Job Postings: Challenges and Best Solutions
How to skyrocket your Marketing Effort?
Some decades ago, playing games was an act associated with childhood and immaturity. Nevertheless, regardless of one’s age, almost everyone you know has probably played a game or two.